The proliferation of AI has rapidly introduced many new software technologies, each with its own potential misconfigurations that can compromise information security. Thus the mission of UpGuard Research: discover the vectors particular to a new technology and measure its cyber risk.
This investigation looks at llama.cpp, an open-source framework for using large language models (LLMs). Llama.cpp has the potential to leak user prompts, but because there are a small number of llama.cpp servers in the wild, the absolute risk of a leak due to llama.cpp is low.
However, in examining the prompt data exposed by poorly secured llama.cpp servers, we found two IP addresses generating text for sexually explicit roleplay involving characters who were children. While our purpose in doing this research was to understand the risk associated with exposed llama.cpp servers, the particular data exposed speaks to some of the most pressing safety concerns associated with generative AI.
Artificial Intelligence and the Future of TPRM
We break down the differences between various AI technologies, explain how AI provides value in the TPRM workspace, and go under the hood of UpGuard’s advanced AI-powered TPRM solution: Vendor Risk.
What is llama.cpp?
Llama.cpp is an open-source project for “Inference of Meta's LLaMA model (and others) in pure C/C++.” In non-technical terms, llama.cpp is free software that makes it easy to start running free AI models. The best-known AI products—like OpenAI’s ChatGPT, Anthropic’s Claude, or Google’s Gemini—use proprietary models accessed through an application created by the owner (like what you see at chatgpt.com) or through APIs that provide paid access to the models. But there are also many free and open-source large language models that users can download, modify, and run in their own environments. Llama is one family of open models released by Meta and the reason so much AI-related software has “llama” in the name. Projects like llama.cpp or Ollama provide the interface to use those models.

Llama.cpp can be configured as an HTTP server with APIs that allow remote users to interact with llama.cpp. If llama.cpp is configured to run a server and exposes its interface to the public internet, then anonymous remote users can detect the llama.cpp server and interact with it. Generally speaking, it is a bad idea to expose APIs to the internet unless they are genuinely intended for everyone in the world to access. However, because llama.cpp is a free and open piece of software, it is commonly used by hobbyists who may not worry about the information security of a toy app in their personal lab.
Those two features– HTTP APIs that can be made publicly accessible and commonly used by individuals outside of corporate governance– increase the likelihood that users will configure llama.cpp servers with less-than-secure settings.
Risks of exposed llama.cpp APIs
To study the real-life prevalence of those risks, we used two llama.cpp GET
APIs: `/props` and `/slots`. Llama.cpp also provides various POST
APIs that we did not attempt to use because of the possibility of disrupting server operations. For a complete security audit of an application using llama.cpp, you should also ensure those POST
APIs cannot be abused.
The /props
endpoint provides metadata about the model parameters. This is similar to the data that Ollama APIs commonly expose. The v1/models
and /health
endpoints provide the same data accessible through /props
, separated out to be OpenAI compatible or to simplify performing a health check, respectively. Because they provide redundant data, we did not bother calling those endpoints.
The /slots
endpoint provides the actual text of the prompts along with metadata about prompt generation. If /slots
is enabled and accessible without authentication, anonymous users can read the messages sent to the model. If anyone is using the model in pretty much any way, exposing their inputs is undesirable. The llama.cpp documentation includes this warning about /slots
: “This endpoint is intended for debugging and may be modified in future versions. For security reasons, we strongly advise against enabling it in production environments.” Good advice, llama.cpp team!
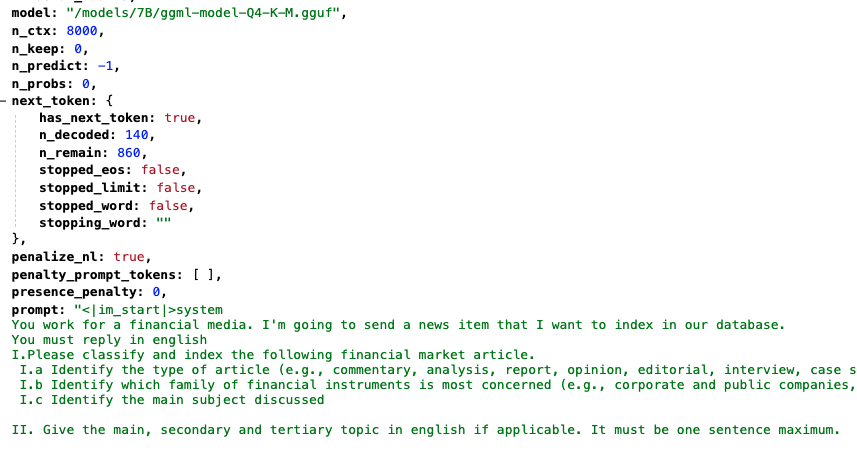
Artificial Intelligence and the Future of TPRM
We break down the differences between various AI technologies, explain how AI provides value in the TPRM workspace, and go under the hood of UpGuard’s advanced AI-powered TPRM solution: Vendor Risk.
Overall prevalence and distribution of llama.cpp
The first thing to note is that there are a relatively small number of llama.cpp servers exposed worldwide—only about 400 at any given time during our research in March 2025. By comparison, the number of exposed Ollama servers is currently around 20k, almost triple what it was two months ago. However, the possibility for anonymous users to read prompts from llama.cpp servers makes them potentially more impactful with lower effort to exploit.
Geographically, the llama.cpp servers are concentrated in the US, with Germany and China clearly in second place. Comparing the distribution of llama.cpp servers to Ollama, llama.cpp is proportionally less common in China and more common in Germany.
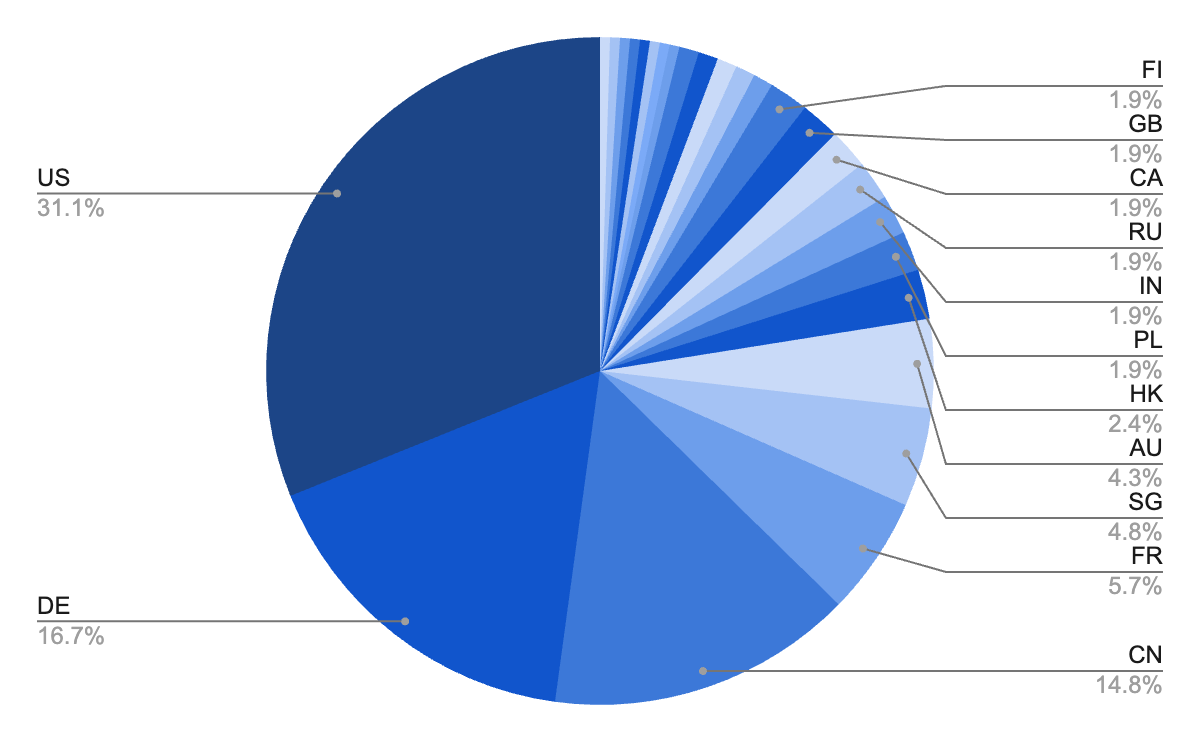
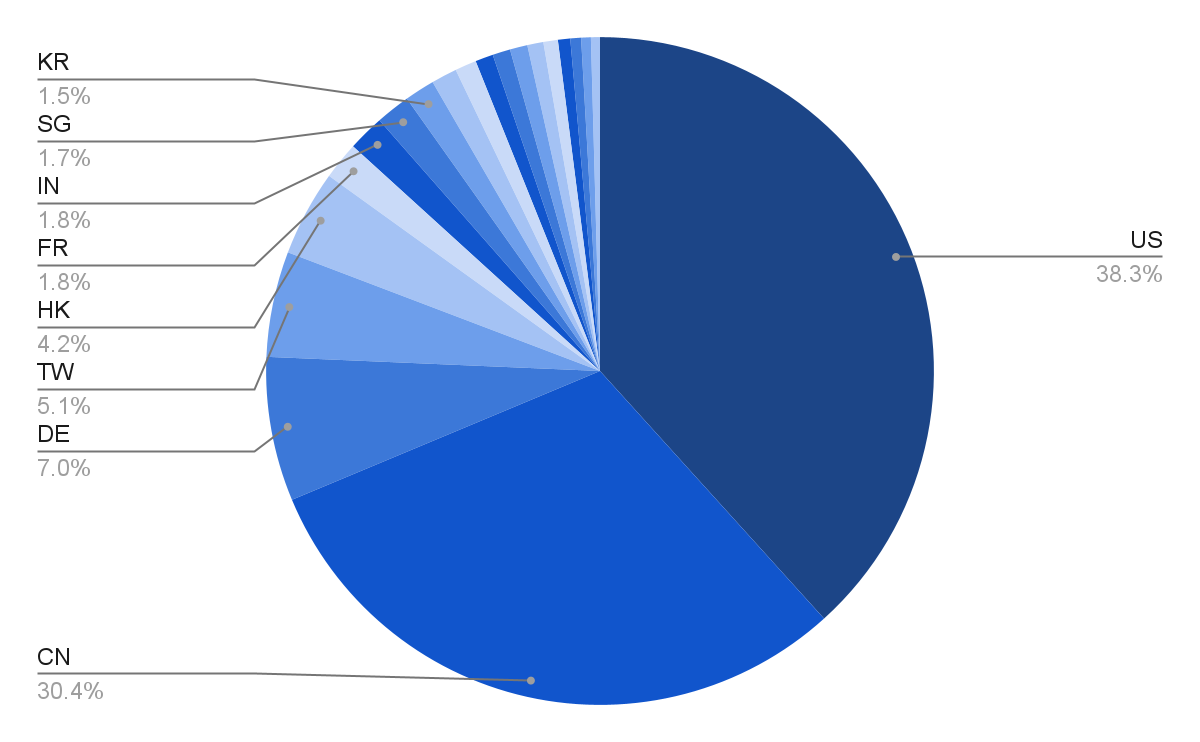
As with Ollama, the organizations owning the IP addresses are diffuse, again indicative of hobbyist users rather than corporate deployments that tend to cluster in major cloud providers like AWS, Azure, and Google.
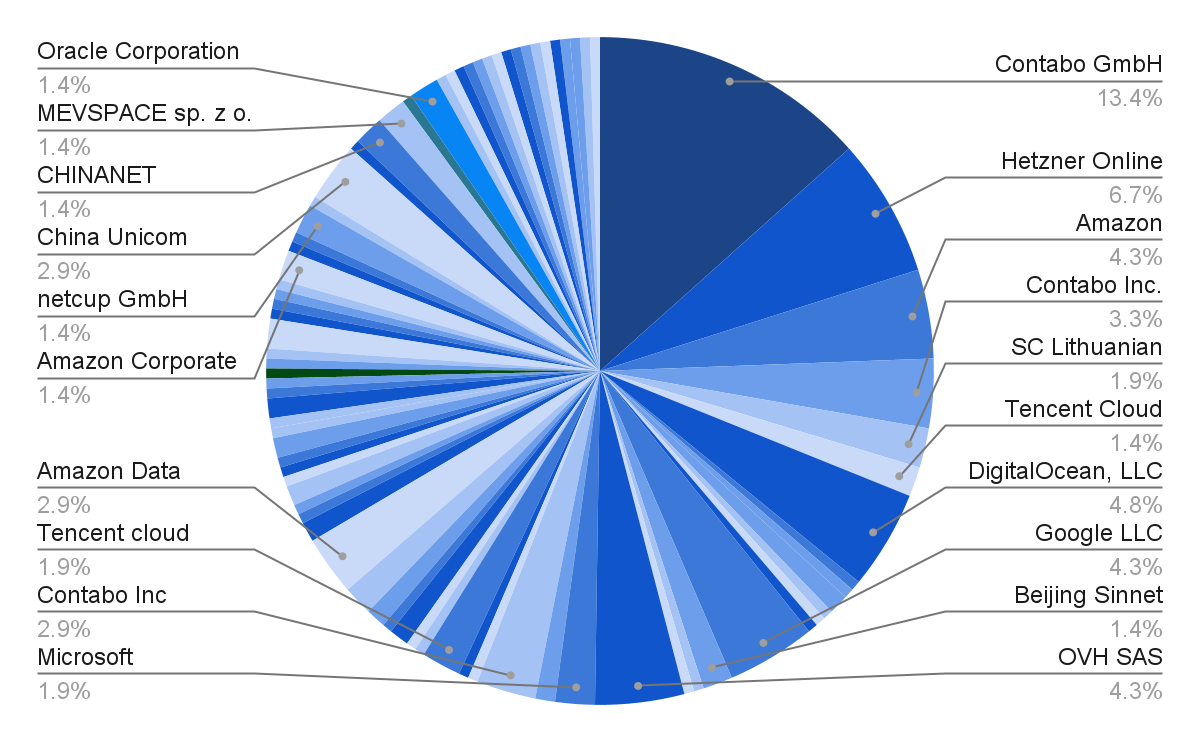
Distribution of /slots and /props endpoints
Given that /slots
exposes more sensitive information than `/props` (and is explicitly warned against in the documentation), we expected to find far fewer instances of /slots
than /props
. While that was the case, the number of llama.cpp instances exposing their prompt data was higher than expected—about one-third of all servers.
Conversely, the number of instances exposing system properties—just over half—is relatively low compared to the thousands of IPs exposing similar data via Ollama APIs.
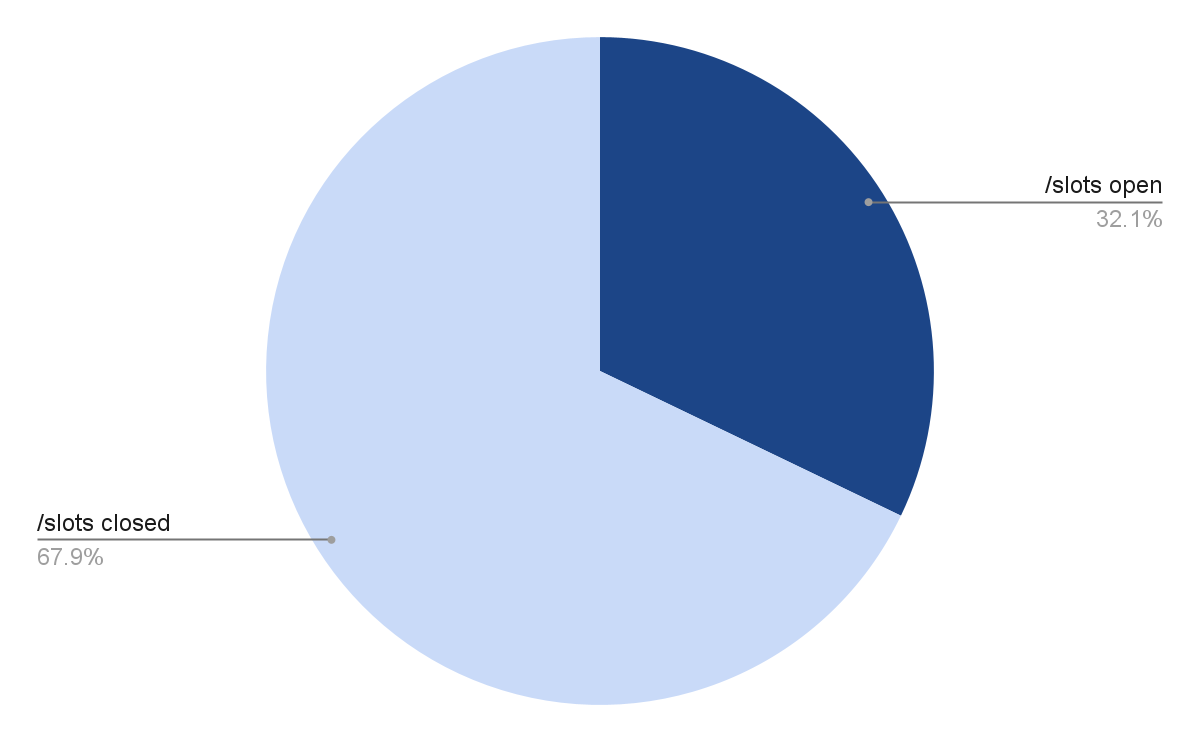

What’s in the prompts?
Of the 117 IP addresses exposing their prompts, very few would qualify as “production” use. Fifty servers showed the last prompt as a default question for testing LLMs, "How does the uncertainty principle affect the behavior of particles?” Forty-four had no recorded prompt at all. A handful had boilerplate analyses of software vulnerabilities, identical prompts to create multiple-choice quizzes, or public documents—evidence of testing the model but not sensitive information, and not in ongoing use.

The llama servers with by far the longest prompts– the ones where the amount of content might indicate “production” use– were three chatbots with prompts for elaborate, long-running, erotic roleplays. Each of those unique IPs used a different model– Midnight-Rose-70B, magnum-72b, and L3.3-Nevoria-R1-70b– indicating they were most likely separate projects that just happened to all share a similar function.
Two of those IPs were frequently updated with new prompts from different scenarios or characters. To measure their activity, we collected the prompts on those IPs from /slots
once per minute for 24 hours. Using a hash of the prompt text, we then deduplicated the prompts for a total of 952 unique messages in that period, or around 40 prompts per hour. We did not submit or interact with the prompting interface, only observed publicly exposed prompts accessible via passive scanning.
After reviewing the data, the major concern was that some of the content created by those LLMs described (fictional) children from ages 7-12 having sex.
To count the number of unique scenarios, we sliced the first 100 characters from each message to get a representative sample of the system prompt for each scenario. This method identified 108 unique system prompts or narrative scenarios. The scenario with the longest chat had 150 messages within the 24-hour period. Of the 108 scenarios, five involved children.

While this content does not involve any real children, the prevalence of AI-generated CSAM in even a narrow slice of this data shows how much LLMs have lowered the barrier to entry to generating such content. Moreover, the content is not just static text but a text-based interactive CSAM roleplay—something that is repellant, if not illegal, and which LLM technology makes trivial to create. Indeed, there are already many sites that operate as platforms for users to create “characters” for “uncensored” roleplay in hundreds of thousands of scenarios.
Conclusion
Information security teams should be aware of llama.cpp as a technology that can be misconfigured and expose confidential data. UpGuard’s cyber risk scanning detects IP addresses running llama.cpp to prevent third-party data breaches. When using llama.cpp, implementers should be familiar with secure configuration guidelines, most notably not enabling the /slots
endpoint. In this survey, we did not detect any exposures of corporate data, but the potential exists, and infosec programs should continue monitoring for such events.
The particulars of the data we found accessible through llama.cpp APIs are not especially relevant to corporate information security teams but are applicable to the broader discussion of AI safety. Empirical observation of actually running LLMs through research surveys will be part of the continued project of understanding AI’s impact on society and what guardrails are needed.